Within our HCI classes, we have started reviewing the UX of an upcoming multi-platform game from a prominent client, and are performing an expert review on it. An expert review, as opposed to a user-based study, involves having usability experts play the game themselves, and uses tools and their expertise to find faults. This is different to a user-based study, where the expert would observe another player playing the game. Because of the time constraints involved, we selected an expert review as the most effective method to review the UX of this game.
To get the best results possible, and be as helpful as possible to the client, we had to choose our methodology carefully. In this blog post, I’ll discuss how we chose to approach this task, why we chose these methods, and what the alternatives are.
The first rule placed on us is that we are to work in groups of 3. As described in an article by Laitinen on performing expert evaluations, the evaluation reaches its optimal group size between 3 and 4. Less experts than this may miss things. More experts than this fail to find a significantly larger number of faults.
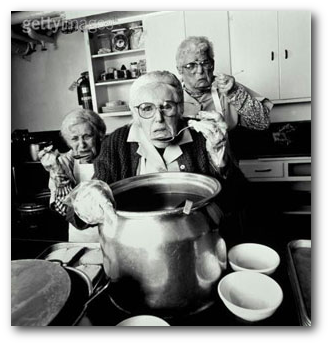
plus too many cooks spoil the broth
The other restraint placed upon us is that we would only have a short amount of time with the game. We decided to use this time to play and evaluate the games separately, and then come together to discuss our findings. The alternatives to this would have been having one person play, and the other two take notes, or to have each person play for a bit (as we did), but the experts not playing would take notes then. All of these sessions would involve filming the game screen, and the participant.
Two experts watching one player
Advantages:
- One longer complete play through, so can see player development
- Experts can ask the player questions during their play session
Disadvantages:
- Only one play through, so difficult to see if issues are common or just for this user
- Questions asked during play through may distract/alter playing experience
Three experts playing together, in turns
Advantages:
- Three sessions played through, so can see reoccurring issues
- Experts can get a greater understanding of the game mechanics through playing it
Disadvantages:
- Players wouldn’t get as far as they would with a long session from one player
- Second and third experts play experience will be biased from the experience of the first
Three experts playing separately
Advantages:
- Each player gets an authentic ‘new player’ experience
- Comparing after can show what issues naturally arose for all
Disadvantages:
- Players wouldn’t get as far as in one long play through
- Have to perform expert evaluation after the game play, rather than during.
Since the sessions were all being recorded, we opted to do the last one, and hence have the ‘purest’ play experience recorded for each. There is, of course, no right answer – many other groups chose different approaches, and I’m sure they found equally valid issues. I’d welcome comments below if anyone has reasons for a preference with how to perform an expert evaluation.
Now having a video of a play test, we are individually analyzing them. I’m approaching it using heuristics, such as those made by Nielsen, Nokia, and the work of Federoff as a guide. Having identified the issues, I will then attempt to rate them by severity – the extent to which they will hinder the user’s enjoyment of the game. Then, in a group session with my team members, we will evaluate which issues we all agreed where particularly prominent and severe, and amalgamate our results, ending up with a list of issues with the game.
We will then have to present our data to the client. I posted before about writing a UX report, but the circumstances for this report will differ – Geographical location, and time constraints mean that this report will be an in-person presentation, with some take-aways. I will blog about these soon….!
Hi there, interesting post about HCI research in the area of gaming.
I also read a paper that discusses another way of conducting HCI research in the area of gaming.
They even call it video game HCI.
They analyze games totally different from other software because they are so different, and the existing HCI approaches show only results in conform to standard HCI concepts (efficiency, freedom of errors and learnability). But these approaches don’t allow you to understand the way video games are used as games, for instance the efficiency in games is most likely not high, because games emphasize challenge and difficulty.
Which I totally agree with, what do you think about this?
In this paper they work out 3 approaches to develop video game HCI.
1. Value theory
2. Activity theory
3. Structural semiotics
Value theory is used to describe the conduct(behavior) in games. Values are seen as beliefs of “what to do” in a context. For instance: “Avatar is healthy” is needed for both playing the game as for progressing the game.
Activity theory is used to further analyze conduct (analyze structure of gameplay activities as well as the decomposition into actions and motivations) using the value theory.
It is used because it places a strong emphasis on value at all levels of its model.
It provides this model which is separated in components which can be thought about separately and in relation to each other. The model used is the Vygotsky triangle.
Structural semiotics are used to analyze differences in values. The model that is used here is the semiotics square. The square aids in moving away from completely binary understandings of concepts and helps to more deeply understand what a concept such as ‘‘success’’ actually
means.
This is in short how they developed this video game HCI.
Do you think this new approach is useful to use, on the same game you already analyzed?
And will it give you better, other findings?
(if you are interested here is the source of the paper:
Barr P., Noble J. , Biddle R., Video game values: Human–computer interaction and games, Internet, 2 Oktober 2006, (http://ac.els-cdn.com/S0953543806001159/1-s2.0-S0953543806001159-main.pdf?_tid=6bbda6f4-407f-11e3-b29c-00000aacb362&acdnat=1383040356_acc4290fad36e931d77abfd088cf9e5c).)